Control and optimization
Participants: Charles-Henri Bruneau, Angelo Iollo, Iraj Mortazavi, Michel Bergmann.
Flow simulations, optimal design and flow control have
been developed these last years in order to solve real
industrial problems : vortex trapping cavities with CIRA
(Centro Italiano Ricerche Aerospaziali), reduction of
vortex induced vibrations on deep sea riser pipes with IFP
(Institut Français du Pétrole), drag reduction of a ground
vehicle with Renault or in-flight icing with Bombardier
and Pratt-Wittney are some examples of possible
applications of these researches. Presently the recent
creation of the competitiveness cluster on aeronautics,
space and embedded systems (AESE) based also in Aquitaine
provides the ideal environment to extend our applied
researches to the local industrial context. There are two
main streams: the first need is to produce direct
numerical simulations, the second one is to establish
reliable optimization procedures. In the next subsections
we will detail the tools we will base our work on, they
can be divided into three points: to find the appropriate
devices or actions to control the flow; to determine an
effective system identification technique based on the
trace of the solution on the boundary; to apply shape
optimization and system identification tools to the
solution of inverse problems found in object imaging and
turbomachinery.
Control of flows
There are mainly two approaches: passive (using passive
devices on some specific parts that modify the shear
forces) or active (adding locally some energy to change
the flow) control. The passive control consists mainly in
adding geometrical devices to modify the flow. One idea is
to put a porous material between some parts of an obstacle
and the flow in order to modify the shear forces in the
boundary layer. This approach may pose remarkable
difficulties in terms of numerical simulation since it
would be necessary, a priori, to solve two models: one for
the fluid, one for the porous medium. However, by using
the penalization method it becomes a feasible task. This
approach has been now used in several contexts and in
particular in the frame of a collaboration with IFP to
reduce vortex induced vibrations.
Another technique we are interested in is to inject
minimal amounts of polymers into hydrodynamic flows in
order to stabilize the mechanisms which enhance
hydrodynamic drag.
The active approach is addressed to conceive, implement
and test automatic flow control and optimization aiming
mainly at two applications : the control of unsteadiness
and the control and optimization of coupled systems.
Implementation of such ideas relies on several tools. The
common challenges are infinite dimensional systems,
Dirichlet boundary control, nonlinear tracking control,
nonlinear partial state observation. The bottom-line to
obtain industrially relevant control devices is the energy
budget. The energy required by the actuators should be
less than the energy savings resulting from the control
application. In this sense our research team has gained a
certain experience in testing several control strategies
with a doctoral thesis (E. Creusé) devoted to increasing
the lift on a dihedral plane. Indeed the extension of
these techniques to real world problems may reveal itself
very delicate and special care will be devoted to
implement numerical methods which permit on-line computing
of actual practical applications. For instance the method
can be successful to reduce the drag forces around a
ground vehicle and a coupling with passive control is
under consideration to improve the efficiency of each
control strategy.
System identification
We remark that the problem of deriving an accurate
estimation of the velocity field in an unsteady complex
flow, starting from a limited number of measurements, is
of great importance in many engineering applications. For
instance, in the design of a feedback control, a knowledge
of the velocity field is a fundamental element in deciding
the appropriate actuator reaction to different flow
conditions. In other applications it may be necessary or
advisable to monitor the flow conditions in regions of
space which are difficult to access or where probes cannot
be fitted without causing interference problems.
The idea is to exploit ideas similar to those at the basis
of the Kalman filter. The starting point is again a
Galerkin representation of the velocity field in terms of
empirical eigenfunctions. For a given flow, the POD modes
can be computed once and for all based on Direct Numerical
Simulation (DNS) or on highly resolved experimental
velocity fields, such as those obtained by particle image
velocimetry. An instantaneous velocity field can thus be
reconstructed by estimating the coefficients ai(t) of its
Galerkin representation. One simple approach to estimate
the POD coefficients is to approximate the flow
measurements in a least square sense.
A similar procedure is also used in the estimation based
on gappy POD. However, these approaches encounter
difficulties in giving accurate estimations when
three-dimensional flows with complicated unsteady patterns
are considered, or when a very limited number of sensors
is available. Under these conditions, for instance, the
least squares approach cited above (LSQ) rapidly becomes
ill-conditioned. This simply reflects the fact that more
and more different flow configurations correspond to the
same set of measurements.
Our challenge is to propose an approach that combines a
linear estimation of the coefficients ai(t) with an
appropriate non-linear low-dimensional flow model, that
can be readily implemented for real time applications.
Shape optimization and
system identification tools applied to inverse problems
found in object imaging and turbomachinery
We will consider two different objectives. The first is
strictly linked to the level set methods that are
developed for microfluidics. The main idea is to combine
different technologies that are developed with our team:
penalization methods, level sets, an optimization method
that regardless of the model equation will be able to
solve inverse or optimization problems in 2D or 3D. For
this we have started a project that is detailed in the
research program.
As for shape optimization in aeronautics, the aeroacoustic
optimization problem of propeller blades is addressed by
means of an inverse problem and its adjoint equations.
This problem is divided into three subtasks:
i) formulation of an inverse problem for the design of
propeller blades and determination of the design
parameters
ii) derivation of an aeroacoustic model able to predict
noise levels once the blade geometry and the flow field
are given
iii) development of an optimization procedure in order to
minimize the noise emission by controlling the design
parameters.
The main challenge in this field is to move from
simplified models to actual 3D model. The spirit is to
complete the design performed with a simplified tool with
a fully three dimensional inverse problem where the load
distribution as well as the geometry of the leading edge
are those provided by the meridional plane analysis. A 3D
code will be based on the compressible Euler equations and
an immersed boundary technique over a cartesian mesh. The
code will be implicit and parallel, in the same spirit as
what was done for the meridional plane. Further
development include the extension of the 3D immersed
boundary approach to time-dependent phenomena. This step
will allow the designer to take into account noise sources
that are typical of internal flows. The task will consist
in including time dependent forcing on the inlet and/or
outlet boundary under the form of Fourier modes and in
computing the linearized response of the system. The
optimization will then be based on a direct approach,
i.e., an approach where the control is the geometry of the
boundary. The computation of the gradient is performed by
an adjoint method, which will be a simple "byproduct" of
the implicit solver. The load distribution as well as the
leading edge geometry obtained by the meridional plane
approach will be considered as constraints of the
optimization, by projection of the gradient on the
constraint tangent plane. These challenges will be
undertaken in collaboration with Politecnico di Torino and
EC Lyon.
Examples: Drag minimization | Lift
maximization | Thrust
generation | Shape
optimization
Vortex Shedding control (POD reduced order model) using synthetic jets.
Drag reduction: convergence towards to stationnary unstable basis flow.
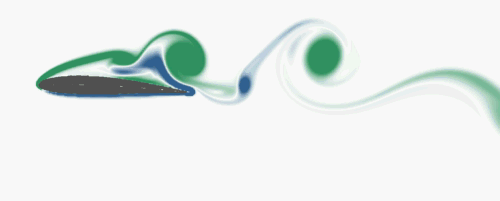
Goal: maximization of the lift coefficient using two flaps.
![]() laminar |
![]() turbulent |
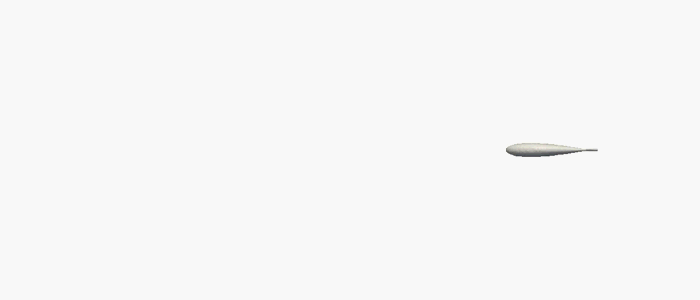
Turbulent fish-like swimming.
Goal: unstationnary shape optimization to generate a thrust force.
Control: tail amplitude to reach a desired swimming velocity.
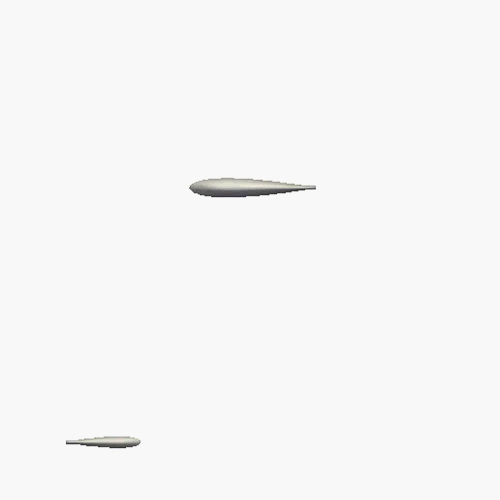
Fish maneuvers.
Goal: large fish sould go towards the small fish
Control: Body deformation law of the large fish by adding adaptive curvature.
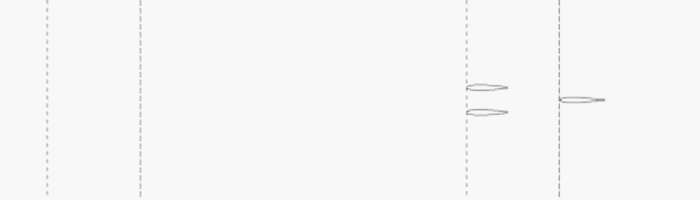
Goal: use the vortices genereted by the 2 leading fishes.
(the 3 fishes have the same simming law)
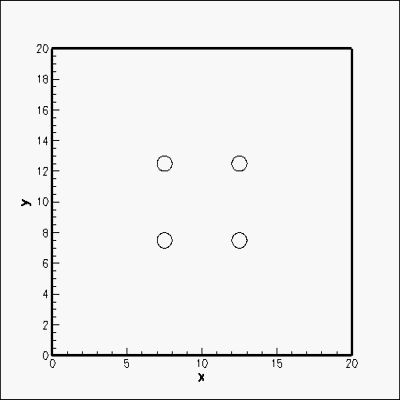
Shape optimization.
Drag reduction in an Euler flow.